The world of Major League Baseball (MLB) prop bets is gaining popularity and expanding at an impressive pace. This increased interest demands a scientific approach to create strategic bets based on reliable data-driven predictions. A crucial statistic used in these predictions is the pitcher’s strikeout rate. However, what happens when the sample size is small, as is often the case at the beginning of the season or when a new player debuts? Bayesian techniques offer a robust solution.
To understand why Bayesian methods are effective, we must first delve into the issue that comes with small sample sizes. Simply put, they lack statistical power. Using early-season performance, for instance, to predict a pitcher’s strikeouts may lead to misleading results due to random variation. A couple of bad or good games can skew the strikeout average, and therefore affect our MLB prop bets.
Here’s where Bayesian techniques come to the rescue. This mathematical framework allows us to incorporate prior knowledge, such as a pitcher’s historical performance or the league average, when making predictions. In essence, Bayesian inference provides a mechanism to blend observed data (the small sample size) with what we already know, thus tempering extreme values that can result from limited data.
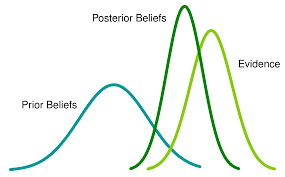
A simple way to apply Bayesian techniques is by using a concept known as “Bayesian shrinkage”. This process “shrinks” raw proportions (like the strikeout rate) towards a pre-set mean. The degree of shrinkage depends on the sample size; smaller samples are shrunk more aggressively. As more data becomes available, the less we rely on our prior beliefs, and the more our estimates are driven by the new data.
To perform Bayesian shrinkage, you start with two things: your prior beliefs about the pitcher’s strikeout rate (usually represented by a Beta distribution), and the observed strikeout rate from the current small sample size. Using Bayes’ theorem, you can then adjust your beliefs based on the observed data. The resulting distribution represents the revised (posterior) beliefs about the pitcher’s strikeout rate.
This method improves early-season predictions of pitcher performance, as it considers a blend of new data and prior beliefs. By using Bayesian techniques, you’re not overly reliant on a small sample size that could be heavily influenced by random variation. Instead, your MLB prop bets are grounded in a more robust, statistically sound prediction.
To optimize Bayesian prediction, you can adjust your priors based on known factors that affect strikeout rates, like a pitcher’s age or injury status. For example, if a pitcher is returning from an injury, we could skew our priors to reflect a likely decrease in performance. As we gather data on the pitcher’s post-injury performance, these priors can be adjusted accordingly. This flexibility is another reason why Bayesian methods are particularly suitable for prediction in dynamic environments like MLB prop bets.
While these techniques require some mathematical sophistication, they’re increasingly accessible thanks to advances in statistical software. Packages like Stan and PyMC3 in Python have made Bayesian inference widely available to data analysts and sports bettors alike. These tools enable you to perform complex Bayesian analyses and generate detailed predictions about pitcher performance.
In conclusion, Bayesian techniques offer a powerful and flexible tool for predicting pitcher strikeouts, especially when the sample size is small. By considering prior knowledge and tempering it with new data, these methods can help bettors make more accurate and informed MLB prop bets. With the rise of data science in sports betting, there’s never been a better time to delve into the world of Bayesian analysis. Whether you’re a seasoned bettor or a sports enthusiast looking for an edge, consider harnessing the predictive power of Bayesian inference in your betting strategy.